About cookies on this site Our websites require some cookies to function properly (required). In addition, other cookies may be used with your consent to analyze site usage, improve the user experience and for advertising. For more information, please review your options. By visiting our website, you agree to our processing of information as described in IBM’sprivacy statement. To provide a smooth navigation, your cookie preferences will be shared across the IBM web domains listed here.
Research objectives
“Resistive memory devices could have a significant influence on future computing systems.”
—Abu Sebastian
ERC Grant recipient
As complementary metal–oxide–semiconductor (CMOS) scaling reaches its technological limits, a radical departure from traditional von Neumann systems, which involve separate processing and memory units, is needed in order to extend the performance of today’s computers substantially. In-memory computing is a promising approach in which nanoscale memory devices, organized in a computational memory unit, are used for both processing and memory. The essential idea is to exploit the physical attributes and state dynamics of these memory devices to compute in place without the need to shuttle the data back and forth between the memory and processing units. The critical element in these novel computing systems is a very-high-density, low-power, variable-state, programmable non-volatile memory device. The overall objective of PROJESTOR was to explore such a memory device as well as to investigate ways in which we can use such memory devices for computational purposes.
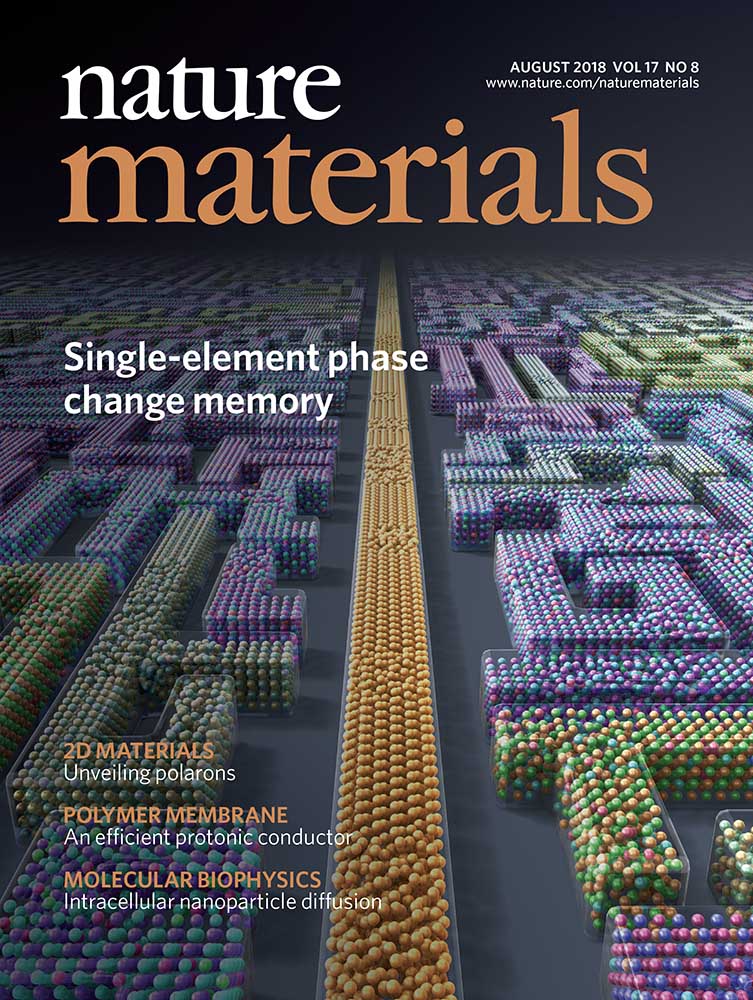
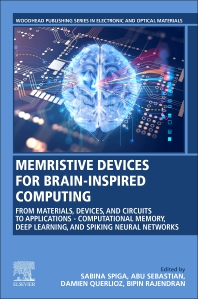
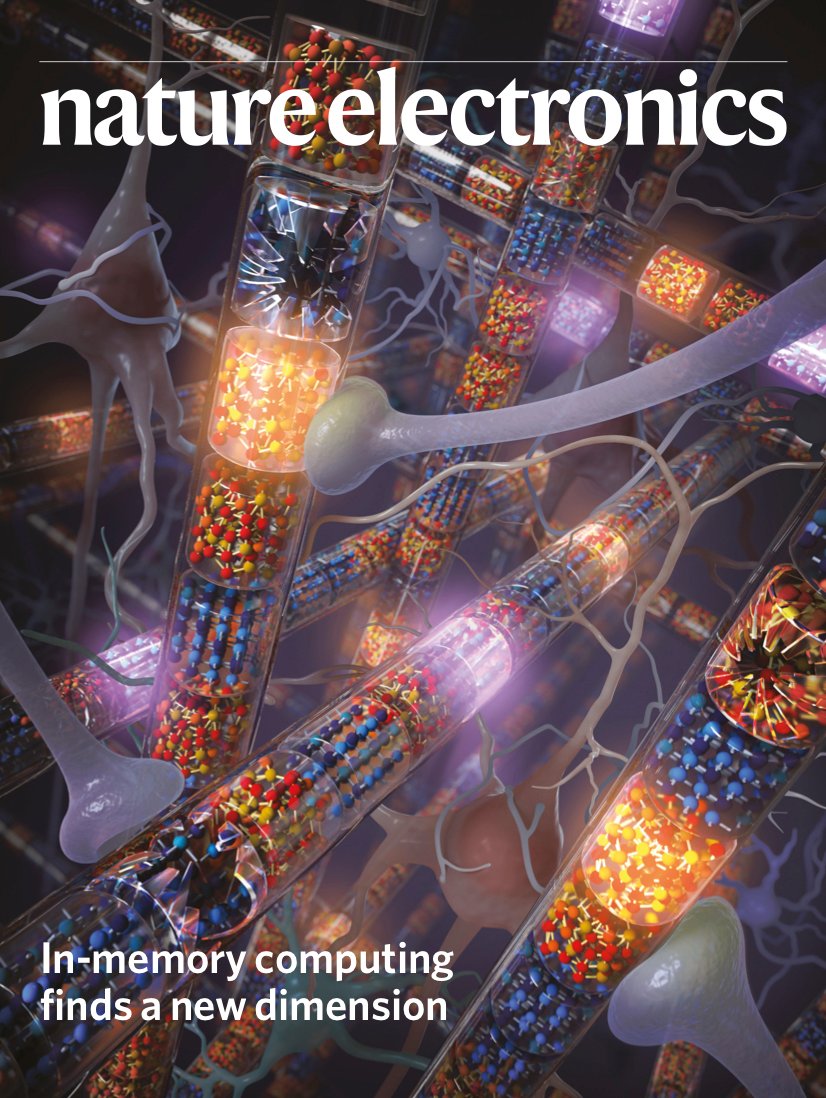
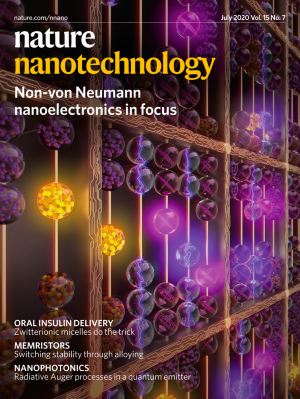
Main achievements
PROJESTOR made critical contributions to both the above-stated goals. On the device front, the project helped establish phase-change memory and in particular projected phase-change memory as a powerful memory device concept for in-memory computing. The key scientific accomplishments in the device front include identification of Antimony as a single elemental phase change memory, significant understanding of structural relaxation in melt-quenched phase-change materials and the demonstration of 8-bit equivalent precision computation using projected phase-change memory. On the application side, a key breakthrough was the concept of mixed-precision in-memory computing which helped expand the application space of analog in-memory computing. We could also identify new applications such as hyperdimensional computing and in-memory database query and experimentally verify using phase-change memory devices.
The first part of the project aimed at developing memory devices for in-memory computing. The goal was to explore the concept of projected memory devices and in particular projected phase-change memory devices where the read and write operations are decoupled by exploiting the nonlinear electrical transport in amorphous phase change materials.
As part of this study, we explored various phase-change materials and projection materials. The most important invention in this front was that of single elemental phase-change materials. We showed how the simplest material imaginable, a single element (in this case, antimony), can become a valid alternative when confined in extremely small volumes. This work appeared on the cover of Nature Materials (August 2018 issue) [1]. We also developed the most comprehensive understanding of resistance drift in phase-change materials, the key attribute that we are trying to counter through the concept of projection. This work was published in Advanced Electronics Materials [2]. More recently, we could experimentally measure the onset of structural relaxation in the melt-quenched amorphous phase [3]. We also established a comprehensive understanding of projected phase change memory [4] and also demonstrated the ability to achieve 8-bit equivalent precision arithmetic operations [5]. The latter work was selected as a highlight paper at IEDM.
In the second part of the project, we explored in-memory computing. We showed several applications where we could exploit the physical attributes and state dynamics of phase-change memory devices to perform computation in place. In one application, we showed how we could perform compressed sensing and recovery. This work was published in IEEE Transactions on Electron Devices [6]. We also demonstrated yet another application where we exploit the crystallization dynamics of phase-change memory devices to solve an unsupervised learning algorithm entirely in the memory [7].
A significant challenge for computational memory is the lack of precision associated with it. We proposed a new concept called mixed precision in-memory computing to address this. We used this concept to solve systems of linear equations and this work appeared in Nature Electronics [8]. The mixed-precision in-memory computing concept was also extended to tackle the challenging problem of training deep neural networks [9]. We also developed a multi-PCM device architecture to counter some of the short comings of in-memory computing [10]. We also explored ways to train networks to be resilient to analog in-memory computing [11]. We also explored other applications such as in-memory hyperdimensional computing [12]. Finally, we wrote a couple of tutorials and review articles that have gained a lot of attention in recent years [13,14].
During the course of the project, we made some significant progress beyond the state of the art in both the development of new memory devices as well as their application in computing. In the memory device front, the exploration of singe elemental phase-change materials is a paradigm shift in this field given that up to now, much of the effort was on fine tuning the material composition. With our work we have shown that, it is essential to focus on nanoscale size effects instead. We also explored projected PCM devices with single elemental phase-change materials as well projected phase change devices based on a mushroom-type device geometry. We also gained a deep understanding of structural relaxation in melt-quenched amorphous phase-change materials. We established the collective relaxation model that quantitatively captures structural relaxation. More recently, we showed that the collective relaxation model could also explain the onset of relaxation that we experimentally measured.
In the computing front, we developed numerous applications for in-memory computing from compressed sensing and temporal correlation detection to solving systems of linear equations. We also showed that we can achieve in-memory scalar operations with projected PCM devices with an equivalent precision of 8-bit fixed point arithmetic and consuming less than 10fJ. This is the first time such high precision is reported using in-memory computing. In the second half of the project, we made critical contributions to in-memory deep learning. We established ways to achieve software-equivalent accuracy training and inference using in-memory computing. We also explored machine learning algorithms that transcend deep learning such as hyperdimensional computing.
ReferencesTeam
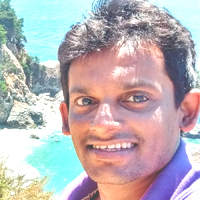
Geethan Karunaratne
Relevant publications (selected)
- G. Bent, C. Simpkin, I. Taylor, A. Rahimi, G. Karunaratne, A. Sebastian, D. Millar, A. Martens, K. Roy,
“Energy efficient 'in memory' computing to enable decentralised service workflow composition in support of multi-domain operations,”
SPIE Proceedings, Publisher: The international society for optics and photonics, 2021 - I. Giannopoulos, A. Singh, M. Le Gallo, V. P. Jonnalagadda, S. Hamdioui, A. Sebastian,
“ -memory database query,”
Adv. Intelligent Systems, 2020 - R. Khaddam-Aljameh, P. A. Francese, L. Benini, E. Eleftheriou,
“An SRAM-based multibit in-memory matrix-vector multiplier with a precision that scales linearly in area, time and power,”
IEEE Transactions on Very Large Scale Integration Systems, 2021 - R. Khaddam-Aljameh, M. Martemucci, B. Kersting, M. Le Gallo, R. L. Bruce, M. BrightSky, A. Sebastian,
“A multi-memristive unit-cell array with diagonal interconnects for in-memory computing,”
IEEE Transactions on Circuits and Systems II: Express Briefs, 2021 (open access) - B. Kersting, S. G. Sarwat, M. Le Gallo, K. Brew, S. Walfort, N. Saulnier, M. Salinga, A. Sebastian,
“Measurement of onset of structural relaxation in melt-quenched phase change materials,”
Advanced Functional Materials, 2021 (open access) - G. Karunaratne, M. Le Gallo, M. Hersche, G. Cherubini, L. Benini, A. Sebastian, A. Rahimi,
“Energy efficient in-memory hyperdimensional encoding for spatio-temporal signal processing,”
IEEE Transactions on Circuits and Systems II: Express Briefs, 2021 - J. Feldmann, N. Youngblood, M. Karpov, H. Gehring, X. Li, M. Stappers, M. Le Gallo, A. Lukashchuk, A. Raja, J. Liu, D. Wright, A. Sebastian, T. Kippenberg, W. Pernice, H. Bhaskaran,
“Parallel convolution processing using an integrated photonic tensor core,”
Nature, 2021 - G. Karunaratne, M. Schmuck, M. Le Gallo, G. Cherubini, L. Benini, A. Sebastian, A. Rahimi,
“Robust high-dimensional memory-augmented neural networks,”
Nature Communications, 2021 - A. Mehonic, A. Sebastian, B. Rajendran, O. Simeone, E. Vasilaki, A. J. Kenyon,
“Memristors - From in-memory computing, deep learning acceleration, and spiking neural networks to the future of neuromorphic and bio-inspired computing,”
Adv. Intelligent Systems, 2020 - G. Karunaratne, M. Le Gallo, G. Cherubini, L. Benini, A. Rahimi*, A. Sebastian*,
“In-memory hyperdimensional computing,”
Nature Electronics, 2020 - S. R. Nandakumar, I. Boybat, M. Le Gallo, E. Eleftheriou, A. Sebastian, B. Rajendran,
“Experimental demonstration of supervised learning in spiking neural networks with phase-change memory synapses,”
Scientific Reports, 2020 - B. Kersting, V. Ovuka, V. P. Jonnalagadda, M. Sousa, V. Bragaglia, Syed Ghazi Sarwat, M. Le Gallo, M. Salinga, A. Sebastian,
“State dependence and temporal evolution of resistance in projected phase change memory,”
Scientific Reports, 2020 - V. Joshi, M. Le Gallo, S. Haefeli, I. Boybat, S. R. Nandakumar, C. Piveteau, M. Dazzi, B. Rajendran, A. Sebastian, E. Eleftheriou,
“Accurate deep neural network inference using computational phase-change memory,”
Nature Communications, 2020 - A. Sebastian, M. Le Gallo, R. Khaddam-Aljameh, E. Eleftheriou,
“Memory devices and applications for in-memory computing,”
Nature Nanotechnology, 2020 - M. Le Gallo, A. Sebastian,
“An overview of phase-change memory device physics,”
J. Phys. D: Appl. Phys., 2020 - S. R. Nandakumar, M. Le Gallo, C. Piveteau, V. Joshi, G. Mariani, I. Boybat, G. Karunaratne, R. Khaddam-Aljameh, U. Egger, A. Petropoulos, T. Antonakopoulos, B. Rajendran, A. Sebastian, E. Eleftheriou
“Mixed-precision deep learning based on computational memory,”
Frontiers in Neuroscience, 2020 - A. Sebastian, M. Le Gallo, E. Eleftheriou,
“Computational phase-change memory: beyond von Neumann computing,”
J. Phys. D: Appl. Phys., 2019 - C. Rios, N. Youngblood, Z. Cheng, M. Le Gallo, W.H.P. Pernice, C.D. Wright, A. Sebastian, H. Bhaskaran,
“In-memory computing on a photonic platform,”
Science Advances, 2019 (open access). - A. Sebastian, M. Le Gallo, G.W. Burr, S. Kim, M. BrightSky, E. Eleftheriou,
“Tutorial: Brain-inspired computing using phase-change memory devices,”
J. Appl. Phys. 124, 111101, 2018 (open access). IBM Research Report RZ 3946. - M. Le Gallo, A. Sebastian, G. Cherubini, H. Giefers, E. Eleftheriou,
“Compressed Sensing With Approximate Message Passing Using In-Memory Computing,”
IEEE Trans. Electr. Dev. 65(10), 2018 (open access). - M. Le Gallo, D. Krebs, F. Zipolli, M. Salinga, A. Sebastian,
“Collective structural relaxation in phase-change memory devices,”
Adv. Electronic Materials, 2018.
PDF - M. Salinga, B. Kersting, I. Ronneberger, V.P. Jonnalagadda, X.T. Vu, M. Le Gallo, I. Giannopoulos, O. Cojocaru-Miredin, R. Mazzarello, A. Sebastian,
“Monatomic phase change memory,”
Nature Materials 17, 681–685, 2018 (Cover)
PDF (open access) - I. Boybat, M. Le Gallo, S.R. Nandakumar, T. Moraitis, T. Parnell, T. Tuma, B. Rajendran, Y. Leblebici, A. Sebastian, E. Eleftheriou,
“Neuromorphic computing with multi-memristive synapses,”
Nature Communications 9, 2514, 2018 (open access).
PDF - M. Le Gallo, A. Sebastian, R. Mathis, M. Manica, H. Giefers, T. Tuma, C. Bekas, A. Curioni, E. Eleftheriou,
“Mixed-precision in-memory computing,”
Nature Electronics 1, 246–253, 2018
arXiv preprint arXiv:1701.04279 (open access)
PDF - A. Sebastian, T. Tuma, N. Papandreou, M. Le Gallo, L. Kull, T. Parnell, E. Eleftheriou,
“Temporal correlation detection using computational phase-change memory,”
Nature Communications 8, 2017.
PDF
Videos
“IBM Scientists Demonstrate In-memory Computing with 1 Million Devices for Applications in AI ”
“ Towards Single-elemental Phase Change Memory”
Research in the news
“In-memory computing using photonic memory devices,” IBM Research Blog, 15 Feb 2019.
“New possibilities in artificial intelligence,” issuu, 8 Jan 2019.
“IBM reveals 8-bit analog chip with phase-change memory,” IEEE Spectrum, 3 Dec 2018.
“Dual 8-bit breakthroughs bring AI to the edge,” IBM Research Blog, 3 Dec 2018.
“Keep it simple: Towards single-elemental phase-change memory,” IBM Research Blog, 24 Jul 2018.
“Novel synaptic architecture for brain inspired computing,” IBM Research Blog, 6 Jul 2018.
“Glassy antimony makes monatomic phase change memory,” Physics World, 25 Jun 2018.
“IBM's new in-memory computing solution makes business AI training faster, easier,” TechRepublic, 18 Apr 2018.
“IBM: Our in-memory computing breakthrough will cut cost of training AI,” ZDNet, 18 Apr 2018.
“IBM scientists demonstrate mixed-precision in-memory computing for the first time; Hybrid design for AI hardware,” IBM Research Blog, 17 Apr 2018.
“Want A 200× Faster and More Energy Efficient Computer? IBM Research Develops Just The Tech,” disruptive, 5 Nov 2017.
“IBM’s Phase Change Memory computer can tell you if it’s raining,” The Register, 31 Oct 2017.
“IBM Demonstrates In-memory Computing with 1M PCM Devices,” HPC Wire, 30 Oct 2017.
“IBM can run an experimental AI in memory, not on processors,” MIT Tech Review, 24 Oct 2017.
“Computational Memory,” IBM Research Blog, 24 Oct 2017.
“IBM scientists demonstrate in-memory computing with 1 million devices for applications in AI,” Phys.org, 24 Oct 2017.
“Keralite in team signalling in-memory computing,” Deccan Chronicle, 17 Oct 2017.
“IBM scientist Abu Sebastian develops future memory and computer paradigms with prestigious European grant,” IBM Research Blog, 20 March 2016.
Conference papers
I. Giannopoulos, M. Le Gallo, V. P. Jonnalagadda, E. Eleftheriou, A. Sebastian, “Temperature compensation schemes for in-memory computing using phase change memory,” Proc. International Conference on Artificial Intelligence Circuits and Systems (AICAS), 2020.
A. Sebastian, I. Boybat, M. Dazzi, I. Giannopoulos, V. P. Jonnalagadda, V. Joshi, G. Karunaratne, B. Kersting, R. Khaddam-Aljameh, S. R. Nandakumar, A. Petropulos, C. Piveteau, T. Antonakopoulos, B. Rajendran, M. Le Gallo, E. Eleftheriou, “Computational memory-based inference and training of deep neural networks ,” Proc. VLSI Symposium, 2019.
I. Giannopoulos, A. Sebastian, M. Le Gallo, V. P. Jonnalagadda, M. Sousa, M. N. Boon, E. Eleftheriou, “8-bit precision in-memory multiplication with projected phase-change memory,” Proc. International Electron Devices Meeting (IEDM), 2018 (highlight paper).
4. M. Le Gallo, A. Sebastian, G. Cherubini, H. Giefers, E. Eleftheriou, “"Compressed sensing recovery using computational memory,” Proc. International Electron Devices Meeting (IEDM), 2017.